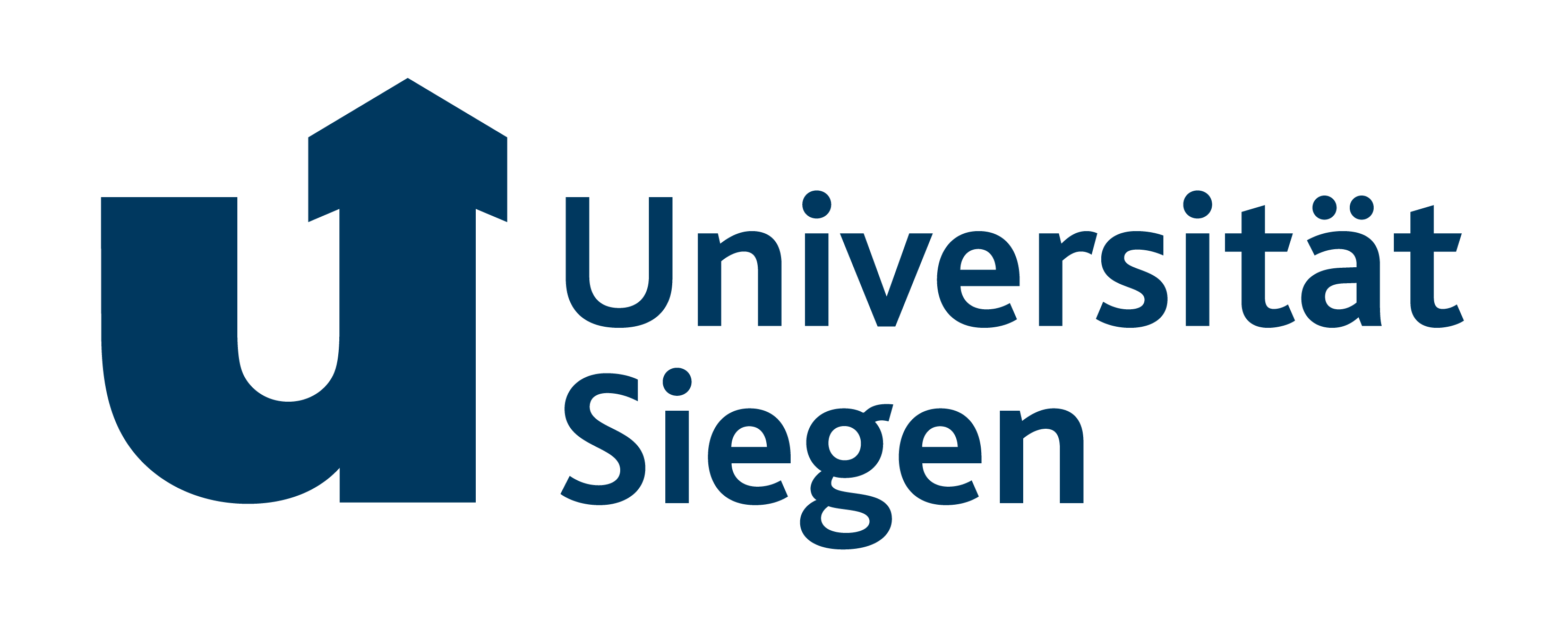
New: Lecture “Compressive Sensing”
Tuesday, 14 -18pm PB-H 0103
Basics:
Classical Shannon sampling theory links the sampling rate to the bandwidth of the signals being acquired. This means that the data flow directly depends on the maximum frequency present in the signal. High-resolution sensors, such as DSLR or mobile phone cameras acquire signals with a very large bandwidth, generating large volumes of data, which needs necessarily to undergo compression for efficient transmission and storage.
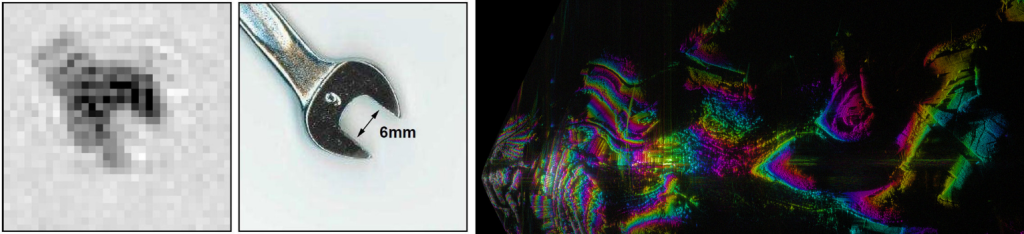
In this lecture we dive into the groundbreaking theory of compressed (or compressive) sensing (CS), which links the sensing effort to the amount of information present in the signal, which does not necessarily depend on its maximum frequency. This way, discrete signals of very high resolution, such as images, can be exactly recovered from a number of measurements that is often well-below that suggested by the Nyquist rate.
Key points of CS:
- Acquire a lower number of more informative measurements.
- Go beyond the limits of Shannon sampling theory (Nyquist rate).
- Link data flow to signal information content.
- Exploit signal sparsity or compressibility in an appropriate domain
(gradient, Fourier, wavelets, etc.). - Integrate a priori knowledge on the signal and the acquisition system to
improve signal reconstruction and/or further reduce the number of
measurements.
Applications:
- Single-pixel Imaging
- Computational Photography
- Imaging for Special Applications (unusual wavelengths)
- Hyperspectral and Multispectral Imaging
- Radar and Remote Sensing
- Time-of-Flight (ToF) and 3D sensing
- Tomography, MRI and other Medical Imaging Techniques
- Communications