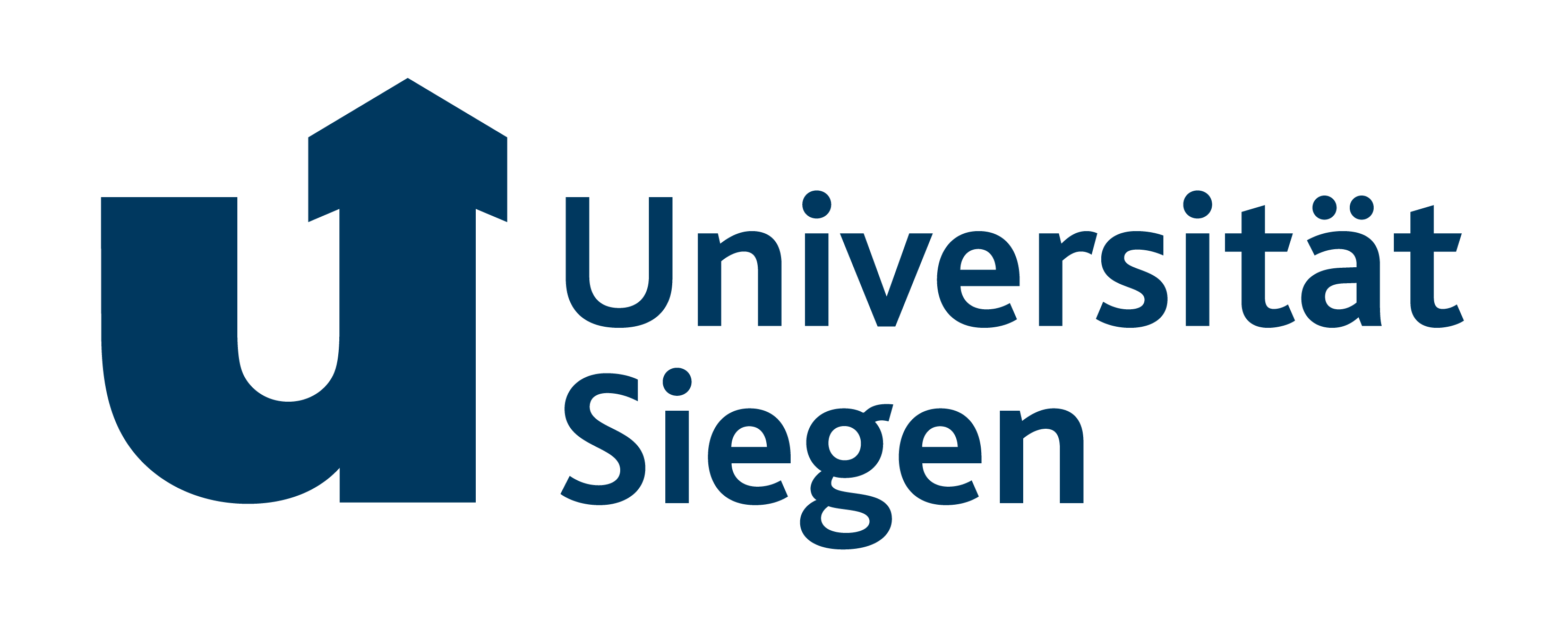
Anna Kukleva (MPI Saarbrücken): Advancing Image and Video Recognition with Less Supervision
14 March 202, 14-15 h in H-C 7327
Deep learning has become an essential component of modern life, transforming various tasks across multiple domains such as entertainment, education, and autonomous driving. However, the increasing demand for data to train models for emerging tasks poses significant challenges. Deep learning models heavily rely on high-quality labeled datasets, yet obtaining comprehensive supervision is resource-intensive and can introduce biases. Therefore, this talk explores strategies to mitigate the need for full supervision and reduce data acquisition costs. The first part of the discussion focuses on self-supervised and unsupervised learning methods, which enable learning without explicit labels by leveraging inherent data structures and injecting prior knowledge for robust data representations. The second part of the presentation discusses strategies such as minimizing precise annotations in multimodal learning, allowing for effective utilization of correlated information across different modalities. Moreover, we discuss open-world scenarios, proposing novel setup and method to adapt vision-language models to the new domains. Overall, this research contributes to understanding learning dynamics and biases present in data, advancing training methods that require less supervision.