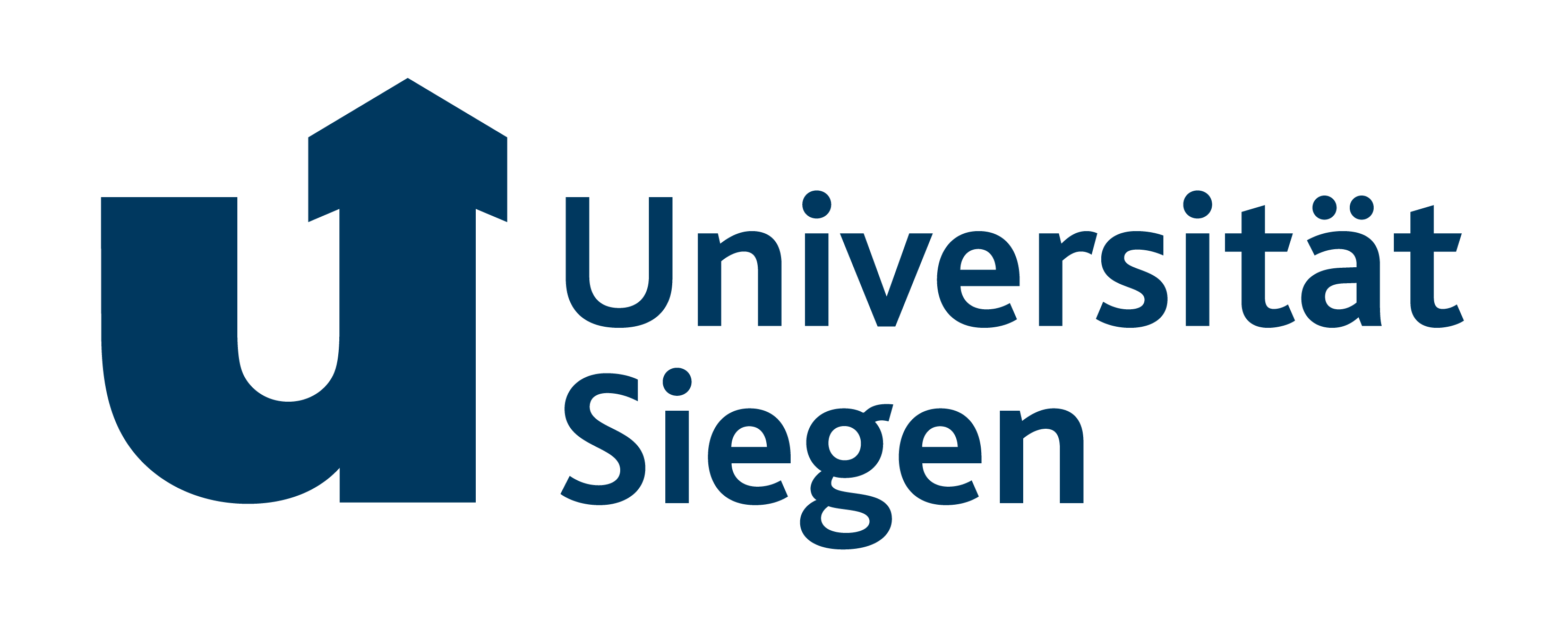
Paolo Zuzolo (University of Bologna): “Deep spectral features for 3D shape analysis”
20th November 2024, 11-12 h in PB-H 0103
3D shape analysis tasks often involve characterizing a 3D object by an invariant, computationally efficient, and discriminative numerical representation, called shape descriptors. Among those, spectral-based shape descriptors have become increasingly widespread, since the spectrum is an isometry invariant, and thus is independent of the object’s representation including parametrization and spatial position[1]. However, large spectral decompositions and the choice of the most significant eigen-couples become computationally expensive for large set of data-points. We introduce a concise learning-based shape descriptor, computed through a Generalized Graph Neural Network (G-GNN) [2]. The G-GNN is an unsupervised graph neural network, leveraging spectral-based convolutional operators, derived from a learnable, energy-driven evolution process. Applied to a 3D polygonal mesh, the G-GNN allows to learn features acting as global shape descriptor of the 3D object. Using a 3D mesh related Dirichlet-like energy leads to a spectral and intrinsic shape descriptor, tied to the isometry invariant Laplace-Beltrami operator. Finally, by equipping the G-GNN with a suitable shape retrieval loss, the spectral shape descriptor can be employed in non-linear dimensionality reduction problems since it can define an optimal embedding, squeezing the latent information of a 3D model into a compact low-dimensional shape representation of the 3D object
[1] Martin Reuter, Franz-Erich Wolter, Niklas Peinecke, Laplace–Beltrami spectra as ‘Shape-DNA’ of surfaces and solids, Computer-Aided Design, Volume 38, Issue 4, 2006, Pages 342-366, ISSN 0010-4485, https://doi.org/10.1016/j.cad.2005.10.011.
[2] D. Lazzaro, S. Morigi, P. Zuzolo, Learning intrinsic shape representations via spectral mesh convolutions, Neurocomputing, Volume 598, 2024, 128152, ISSN 0925-2312, https://doi.org/10.1016/j.neucom.2024.128152.
Paolo Zuzolo received the M.S. degree in Mathematics with the University of Bologna, Bologna, Italy in 2020, and he is currently a Ph.D. student in Mathematics with the University of Bologna, Italy. After completing his master, with a thesis on processing and visualization of astrophysical data, he worked as researcher and developer at CINECA, Bologna, Italy, at the Visualization Information Technology Laboratory. His research interests include numerical methods for inverse problems in image and geometry processing and Geometric Deep Learning.